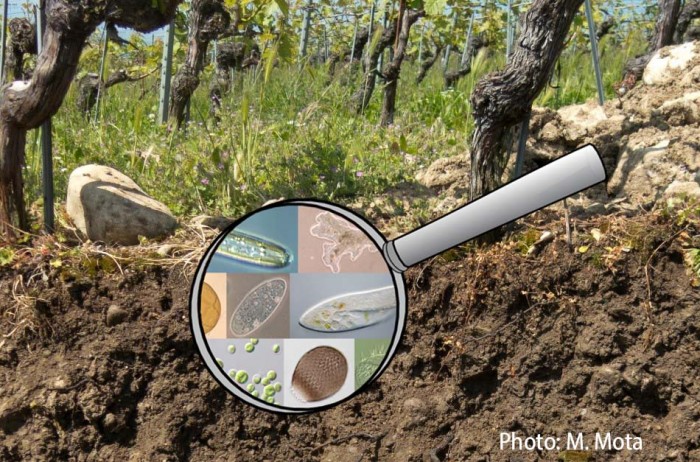
High-throughput sequencing, bioinformatics and machine learning as a soil health diagnostic tool
The impact of farming practices and use of pesticides on soil quality is a growing concern among consumers, farmers and soil managers. To assess this impact, bioindicators, such as protists, have great potential, but their use remains limited because current methods do not allow detailed and rapid analysis of soil samples. To overcome these drawbacks, species identification based on DNA sequences (“barcoding”) coupled with new ultra-high throughput sequencing techniques represents a promising approach. However, the enormous amount of sequences and their high complexity makes it difficult to process them by conventional means. It is therefore essential to develop methods combining bioinformatics and Machine Learning (ML) to (i) quantify, analyze and process protist sequences; (ii) identify and select bioindicators (a subset of protists) associated with different stressors; but also to (iii) model their relative abundance under different conditions, leading to the development of predictive diagnostic models.
We analyzed the composition of protist communities in 28 vineyards in Valais using metabarcoding and compared the predictive performance of different ML algorithms for several variables characterizing soil quality. Our innovative results show that the composition of protist communities can be used to predict a wide range of variables, including the presence of pesticides (copper) in soils. Taxonomically, the protist groups with the highest number of bioindicators were Ciliophora and Cercozoa. Functionally, the majority of bioindicators corresponded to heterotrophic taxa, but some variables (plant biomass and soil pH) were mainly predicted by photosynthetic taxa. Our analyses allowed us to develop scripts to identify biomarkers and predict different soil parameters.
Valorisation
PEÑA C.-A., BROCHET X., FOURNIER B., HEGER T., Quantitative monitoring of agricultural soils using protist communities, SIB day 2020, 8 – 10 juin 2020, Lausanne, Suisse
HEGER T. J., JIBRIL M., STEINER M., XAVIER B., LAMY F., MOTA M., NOLL D., BACHER S., PENA C., Protist communities in vineyard soils: what do they tell us about soil quality and health? Joint meeting of the phycological society of America and the international society of protistologists, 29 juillet – 2 août 2018, Vancouver, Canada
MAMMERI J., BROCHET X., HEGER T., BACHER S., STEINER M., PENA C., MaLDIveS: Machine Learning Diagnostic Soil. SIB days 2018 (Swiss Institute of Bioinformatics), 26-27 juin 2018, Lausanne, Suisse
2017 – in progress
Partner: HEIG-VD
Funding: HES-SO
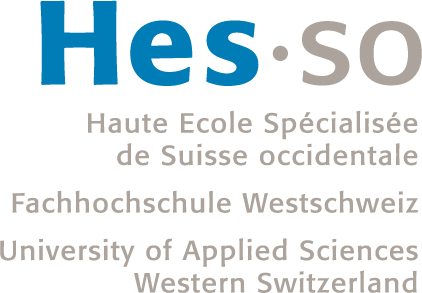
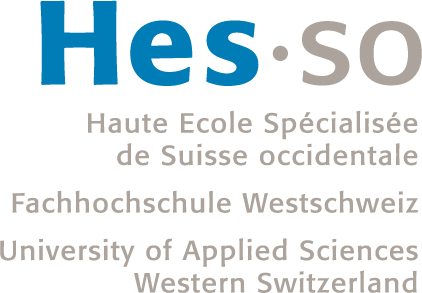